Design test scenarios#
Scenarios offer three types of steps for testing various aspects of a Dataiku project:
Tip
You can review these three sections in any order depending on your needs.
Test a Flow#
Any change in a recipe can cause an unexpected change somewhere downstream in the Flow. To ensure that the Flow is still performing as expected before moving it to a production environment, Dataiku provides an integration test step in a scenario.
Craft test datasets#
Ideally, this kind of testing requires crafting reference datasets that cover as many relevant processing cases as possible. This can be a time consuming process, but it ensures the relevance of the result. Without such reference datasets, having test scenarios may provide a false sense of security.
Navigate to the Flow to inspect the datasets in the Test reference inputs & outputs Flow zone.
Observe the relationship between the following Flow datasets and their test references:
Flow dataset |
Test reference |
---|---|
unlabelled_customers |
test_input_unlabelled_customers |
revenue_loss |
test_reference_revenue_loss |
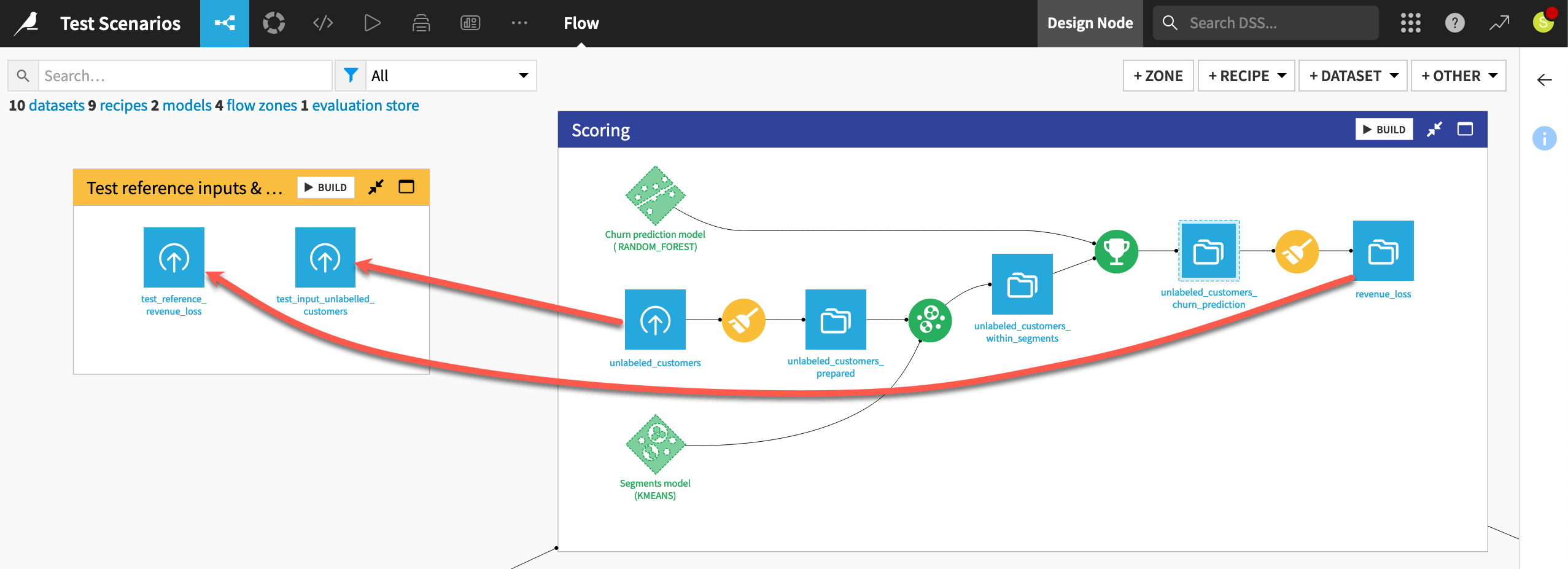
Important
In this case, we have taken small samples as reference datasets. This may be a good way to get started. However, as you progress, the best practice is to specifically craft these datasets.
Create a test scenario#
The project already includes a scenario that we can use for a Flow integration test. However, it hasn’t yet been designated as a test scenario, and so won’t appear in the project’s test dashboard (which we’ll see later). Let’s do that now.
From the Jobs menu of the top navigation bar, select Scenarios.
Open the scenario
Flow Test
.On the Settings tab of the new scenario, check the box Mark as a test scenario.
Navigate to the Steps tab.
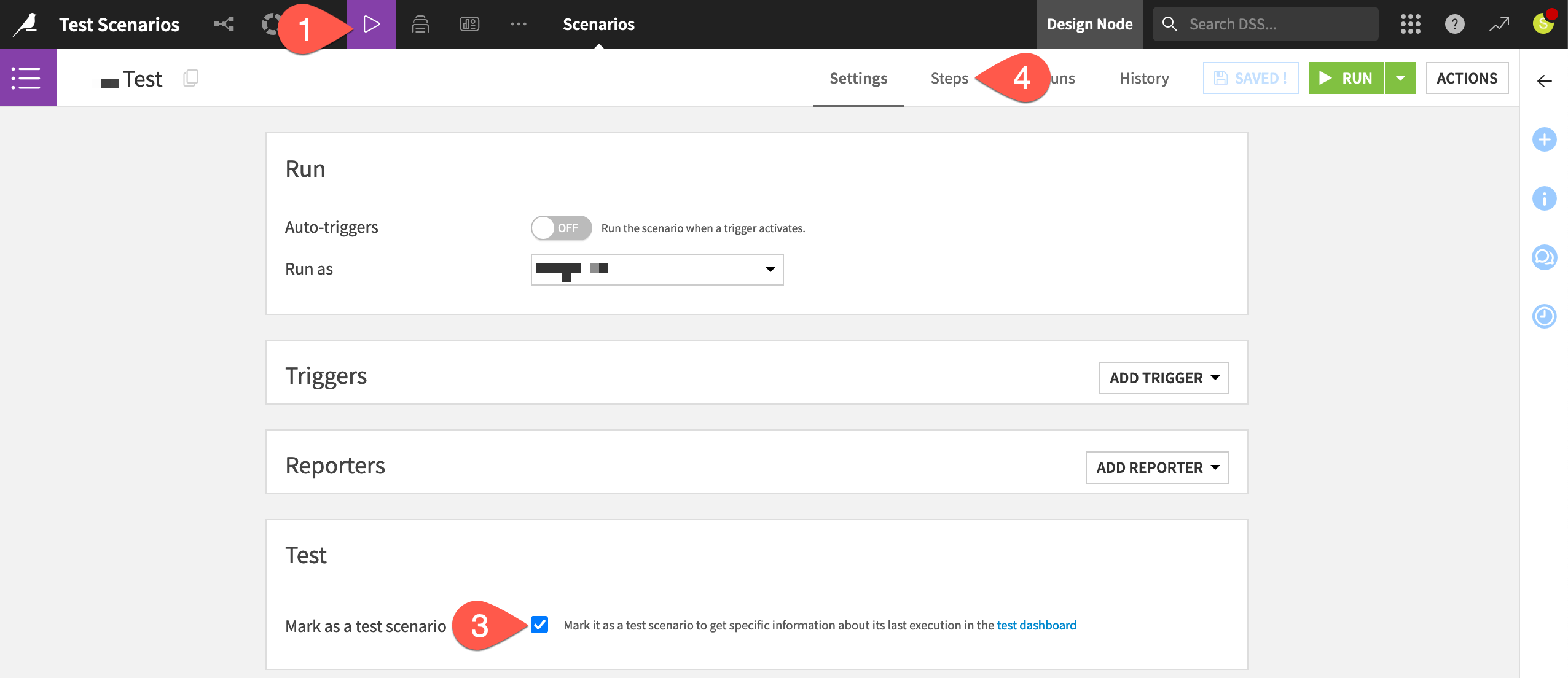
Configure the integration test step#
The integration test step has three key parts to understand:
To be configured |
Purpose |
---|---|
Reference input(s) |
The crafted test dataset(s) that will be used instead of the current input in the Flow. |
Build action(s) |
The Dataiku item(s) in the Flow that the scenario will build (as in an ordinary scenario). |
Reference output(s) |
Output dataset(s) to validate the results. Using the new reference input, the scenario will run the requested build, creating a new output dataset. It will then check if this new output matches the reference output. |
To see this in action, let’s choose an input dataset at the start of the pipeline and an output dataset at the end.
Click Add Step > Run integration test.
Under Reference inputs, click + Add Remapping.
For the current input, select unlabeled_customers.
For the reference input, select test_input_unlabelled_customers.
Under Builds, click + Add Item.
Select the dataset revenue_loss.
Click Add Item.
Under Results validation, click + Add Remapping.
For the current output, select revenue_loss.
For the reference output, select the dataset test_reference_revenue_loss.
Click Save, but don’t yet run it.
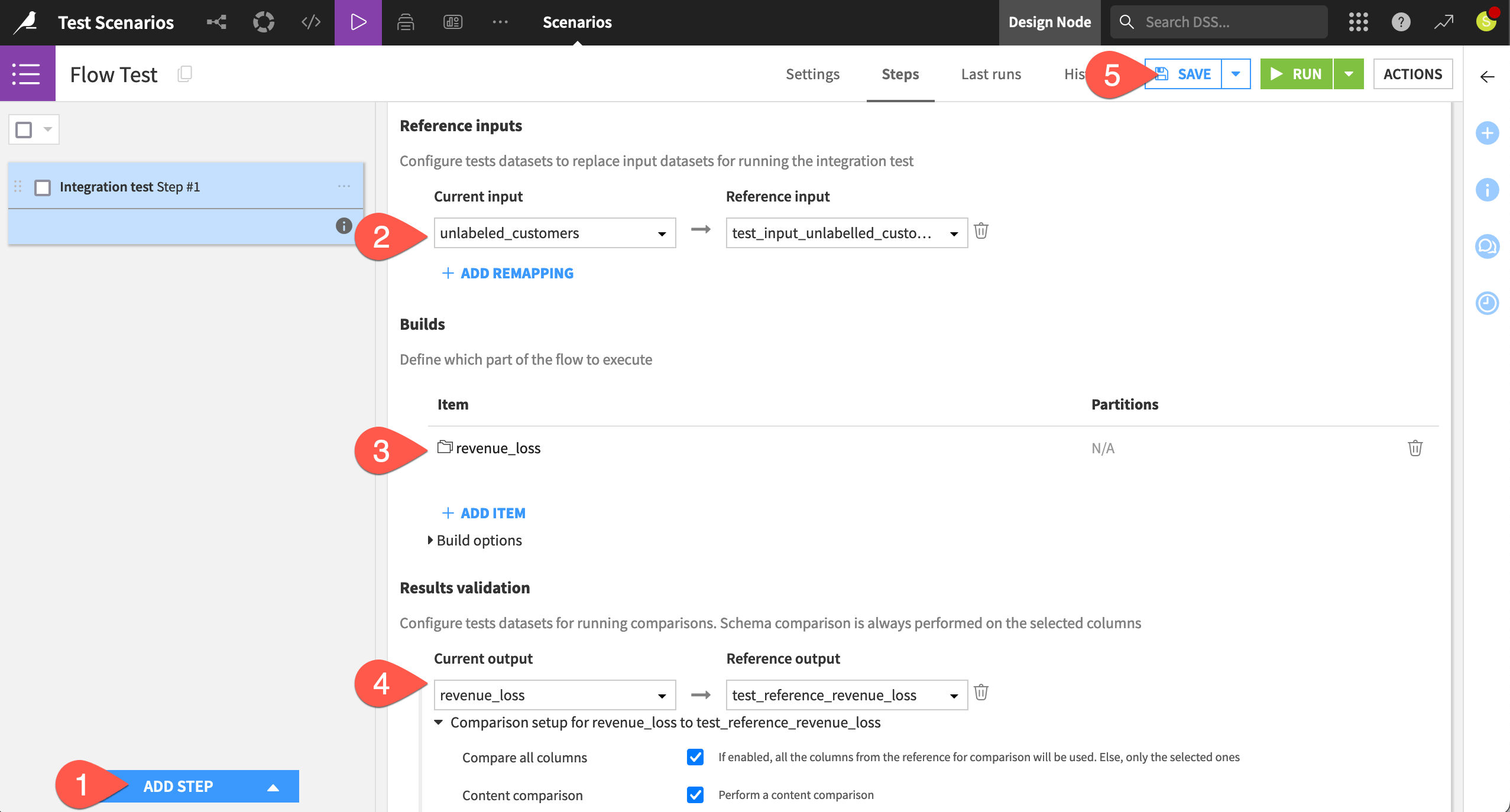
Tip
In this case, we’ve chosen to perform a content comparison across all columns. Depending on the nature of the tests, and therefore the shape of the corresponding reference datasets, you may only want to test a selection of columns.
Run the integration test scenario#
Before running the scenario, let’s introduce an arbitrary Flow change to show how the test would detect it.
Go to the Flow (
g
+f
).Open the Prepare recipe that outputs the revenue_loss dataset.
Make and save a change to the Formula step to create an output that the reference dataset won’t account for, such as:
prediction * ( Total_Charge - (proba_1 * Total_Charge))
Return to the Flow Test scenario, and click Run.
Go to the Last runs tab, and see the failure.
Click View step log to see the reported problem.
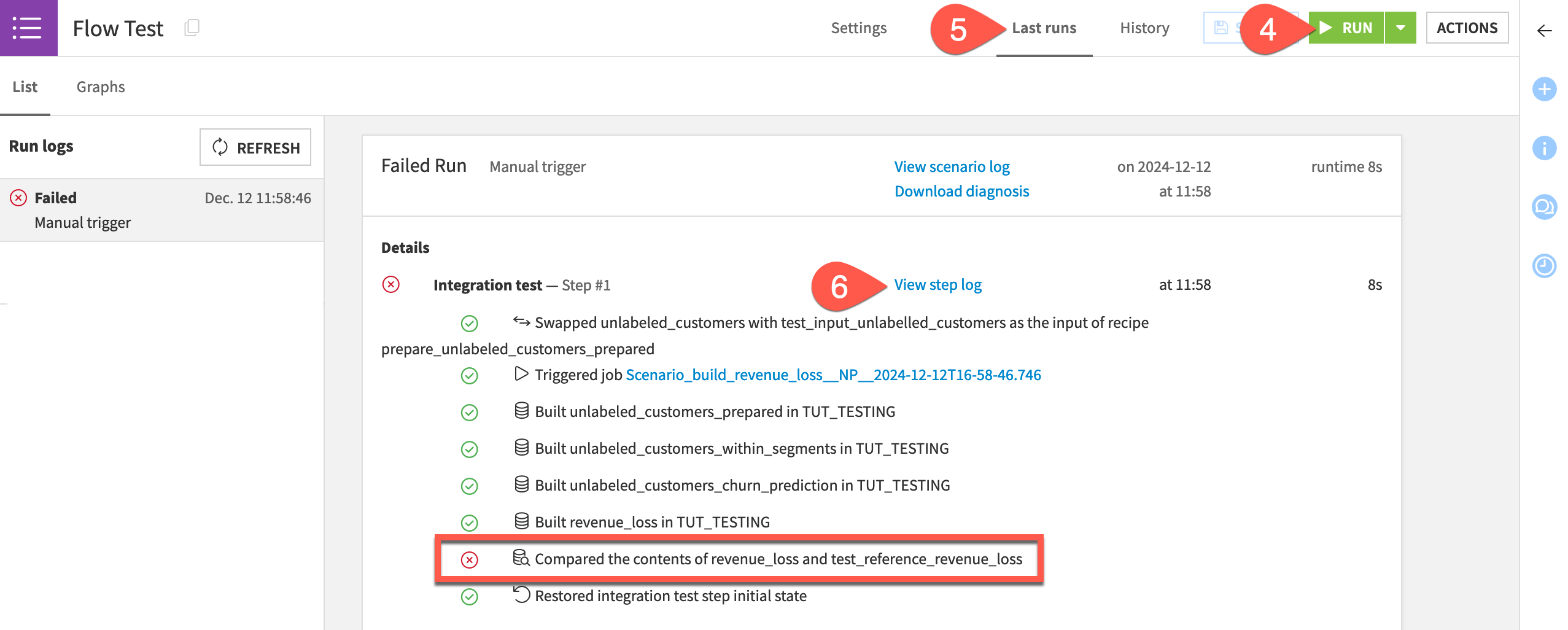
Let’s review the scenario’s activities in detail:
The scenario swapped an upstream input (unlabeled_customers) with a new test input (test_input_unlabelled_customers).
It built an output (revenue_loss) using the new test input.
It compared the new output (revenue_loss) to the provided reference output (test_reference_revenue_loss).
Due to the change in the Prepare recipe, this comparison failed.
Tip
Feel free to fix the cause of the failing test scenario and confirm it succeeds. Note though that having at least one failing test scenario will be useful before moving to the deployment stage of the tutorial.
Test a Python library#
Python users will be familiar with the pytest testing framework. You can use the same framework for unit tests in Dataiku.
Store tests in a project library#
The first step is writing Python unit tests according to the pytest framework and making them accessible from the project’s code library. This step has already been done for you.
From the Code menu of the top navigation bar, select Libraries (or
g
+l
).Explore the sample code in the
python/
folder.
Tip
See the Developer Guide on Project libraries to get started working with code in this way.
Create a test scenario#
Once the actual tests are in place, the next step is having a scenario execute them. An empty scenario has been started for you, but it’s not yet a test scenario.
From the Jobs menu of the top navigation bar, select Scenarios.
Open the Python Test scenario.
On the Settings tab of the new scenario, check the box Mark as a test scenario.
Navigate to the Steps tab.
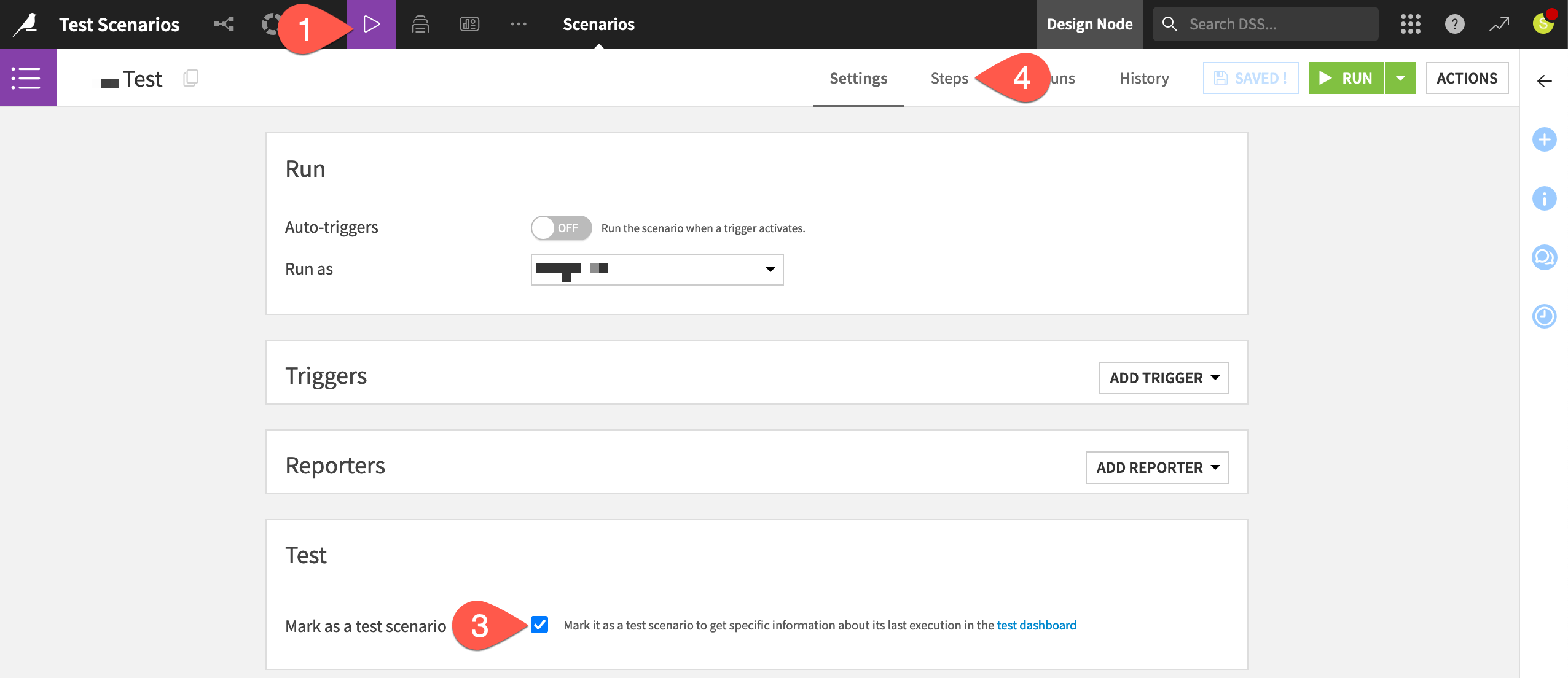
Configure the Python test step#
Next, add the dedicated step to execute the selected Python tests whenever this scenario executes.
Click Add Step > Execute Python test.
Specify the unit tests to run with a pytest selector. In this case, give the folder containing all your tests, which is
python/test
.Select a code environment that includes the pytest library.
Click Run.
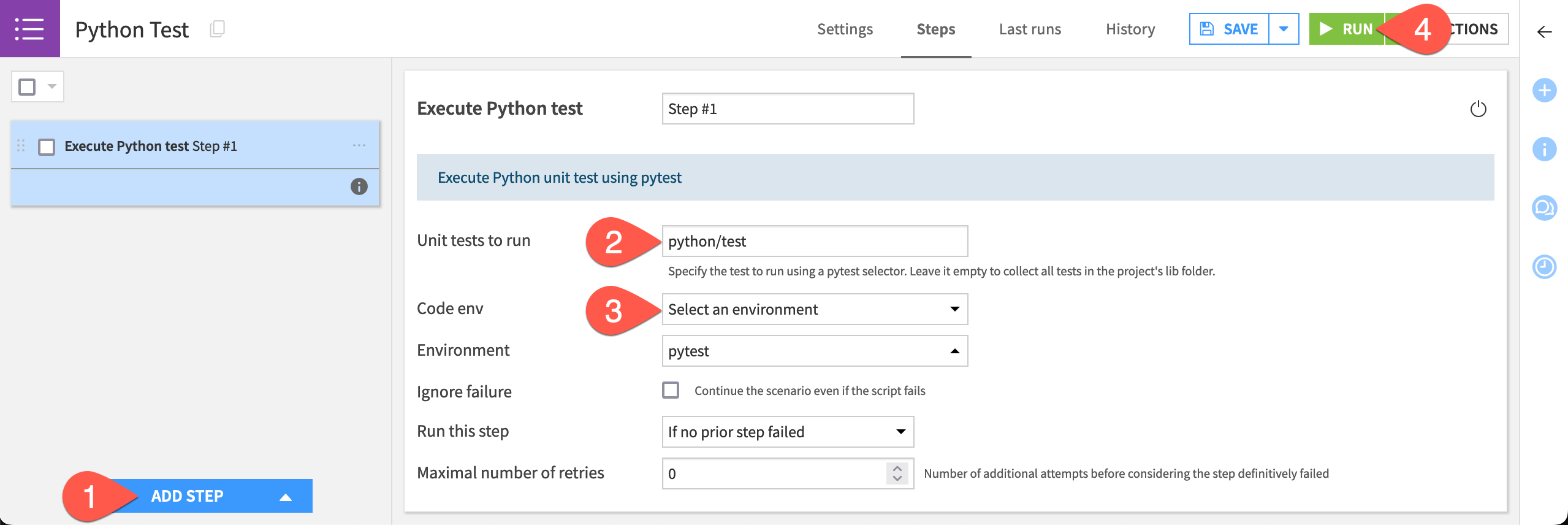
Run the Python test scenario#
Let’s demonstrate both success and failure.
Navigate to the Last runs tab to review the scenario’s progress. The run you’ve just triggered should have succeeded.
Return to the
python/test/test_drift_functions.py
file in the project library.Make and save any edit to introduce a failed test. For example, invert the check in the
test_drift_integer_option_percent
function so that it reads:assert avg_drift_percent > max_drift_percent_expected
Return to the Python Test scenario, and click Run.
View the failure in the Last runs tab.
Click Show log tail or View scenario log to find the cause of the failure.
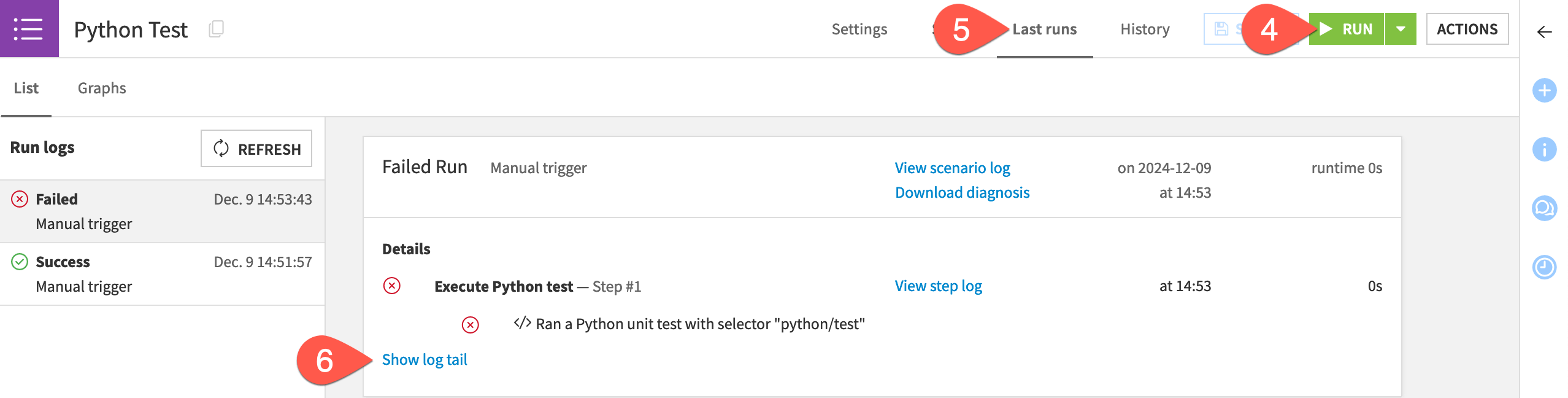
Tip
Feel free to fix the cause of the failing test scenario and confirm it succeeds. Note though that having at least one failing test scenario will be useful before moving to the deployment stage of the tutorial.
Test a webapp#
Webapps can also be tested. No reference dataset or self-written tests are required. This type of step checks whether the webapp is able to start and respond to a ping.
Create a test scenario#
As with the Python test, an empty scenario has been started for you, but it’s not yet a test scenario.
From the Jobs menu of the top navigation bar, select Scenarios.
Open the Webapp Test scenario.
On the Settings tab of the new scenario, check the box Mark as a test scenario.
Navigate to the Steps tab.
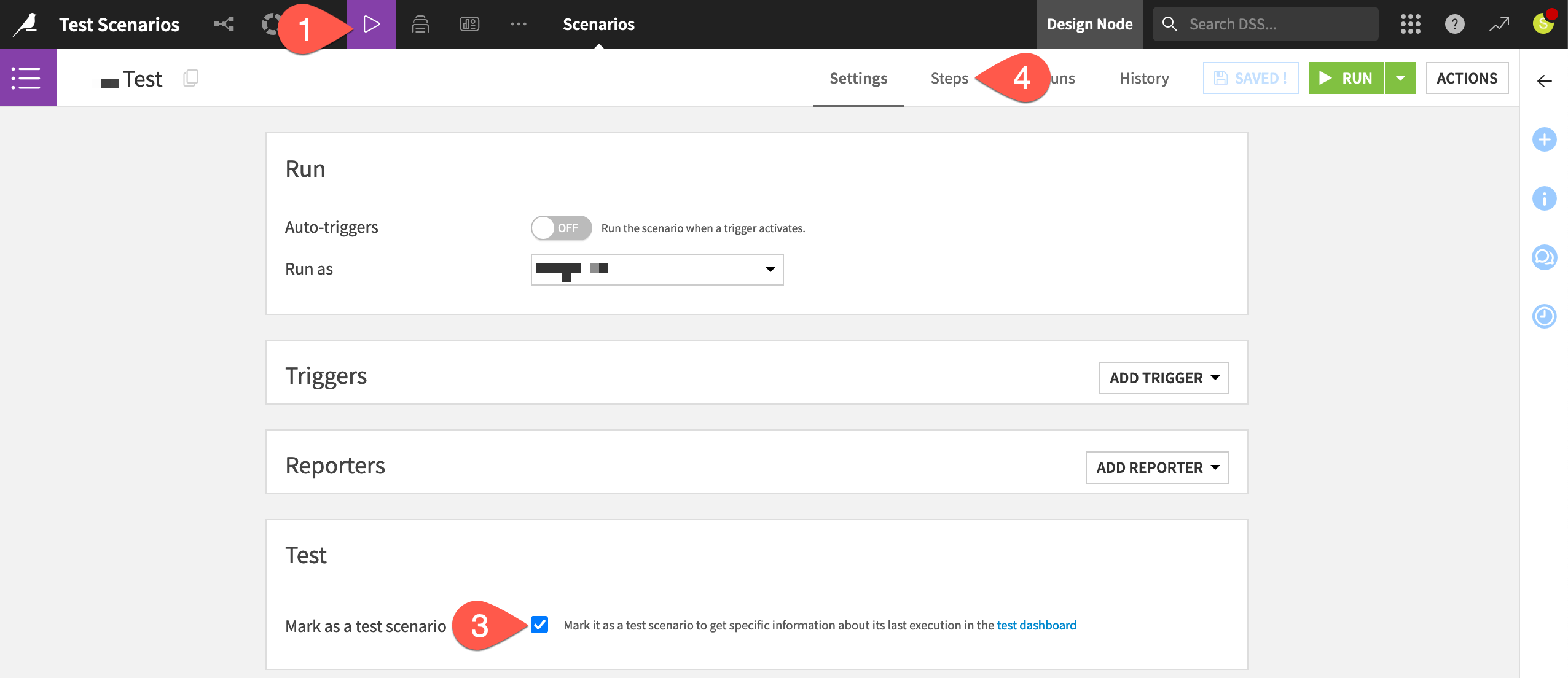
Configure the webapp test step#
Next, add a step to start and ping the webapp whenever this scenario executes.
Click Add Step > Test Webapp.
For Webapp to test, select WebApp1.
Click Run.
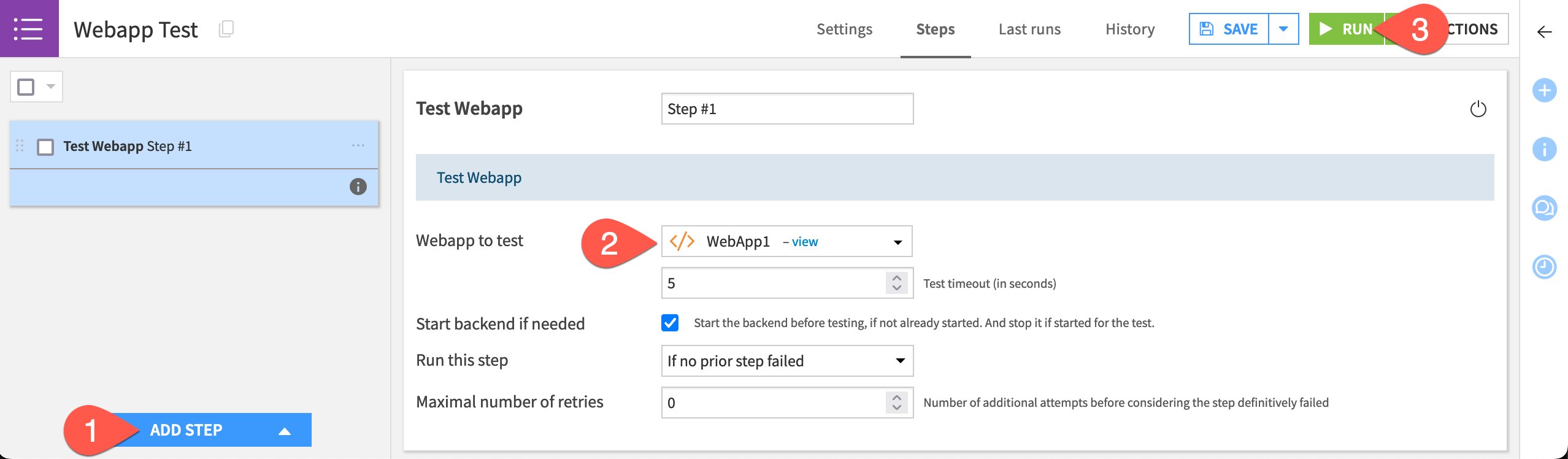
Run the webapp test scenario#
Let’s demonstrate both success and failure.
Navigate to the Last runs tab to review the scenario’s progress. It should succeed.
Open WebApp1, and go to the Settings tab.
Make and save a change that will cause a failure. For example, in the Python tab, uncomment the line to introduce invalid syntax.
Return to the Webapp Test scenario, and click Run.
View the failure in the Last runs tab.
Click View step log to find the cause of the failure.
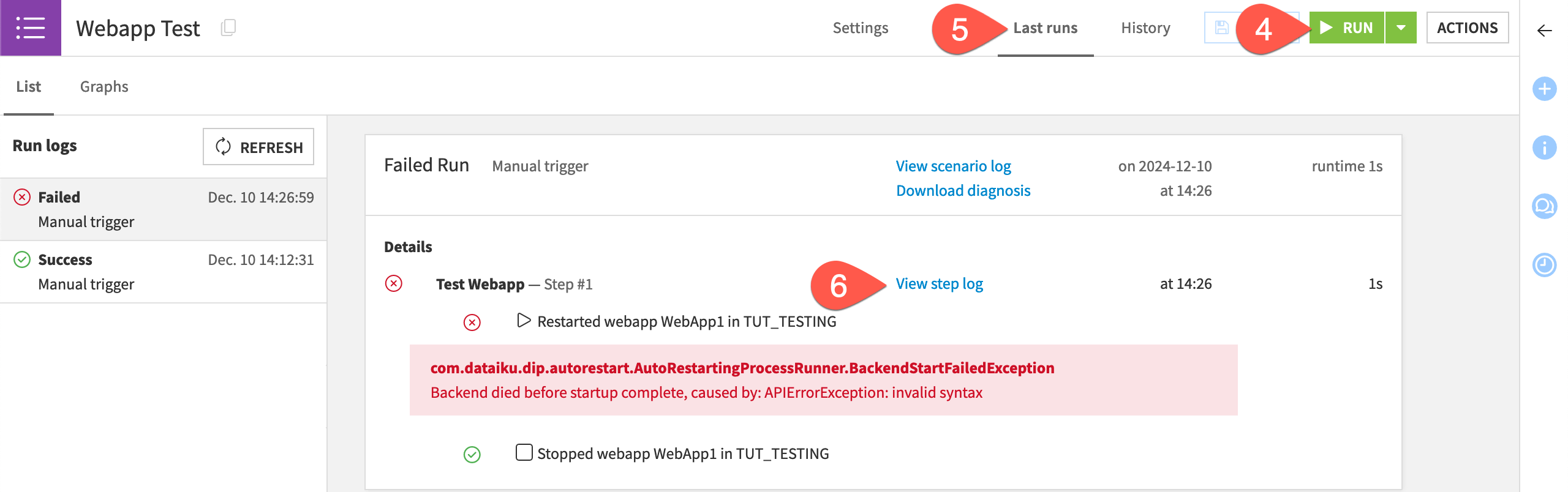
Tip
Feel free to fix the cause of the failing test scenario and confirm it succeeds. Note though that having at least one failing test scenario will be useful before moving to the deployment stage of the tutorial.