Building a first model#
Now that our target variable is built, we need to create the complete train set that will store a list of features as well. The features are the variables we’ll use to try to predict churn.
Let’s create a new SQL recipe, with the train and events_complete datasets as inputs, and a new SQL dataset named train_enriched as output. The recipe is made of the following code:
SELECT train.*
, nb_products_seen
, nb_distinct_product
, nb_dist_0 , nb_dist_1, nb_dist_2
, amount_bought , nb_product_bought
, active_time
FROM train
LEFT JOIN (
-- generate features based on past data
SELECT user_id
, COUNT(product_id) AS nb_products_seen
, COUNT(distinct product_id) AS nb_distinct_product
, COUNT(distinct category_id_0) AS nb_dist_0
, COUNT(distinct category_id_1) AS nb_dist_1
, COUNT(distinct category_id_2) AS nb_dist_2
, SUM(price::numeric * (event_type = 'buy_order')::int ) AS amount_bought
, SUM((event_type = 'buy_order')::int ) AS nb_product_bought
, EXTRACT(
EPOCH FROM (
TIMESTAMP '2014-08-01 00:00:00' - MIN(event_timestamp)
)
)/(3600*24)
AS active_time
FROM events_complete
WHERE event_timestamp < TIMESTAMP '2014-08-01 00:00:00'
GROUP BY user_id
) features ON train.user_id = features.user_id
This query adds several features to the train dataset (containing the target variable). An intuition is that the more a person is active, the less likely she is to churn.
We hence create the following features for each user:
total number of products seen or bought on website
number of distinct product seen or bought
number of distinct categories (to have an idea of the diversity of purchases of a user)
total number of products bought
and total amount spent
Once this first limited set of features is created, our training set is ready. As in the Machine Learning Basics course, create a baseline model to predict the target:
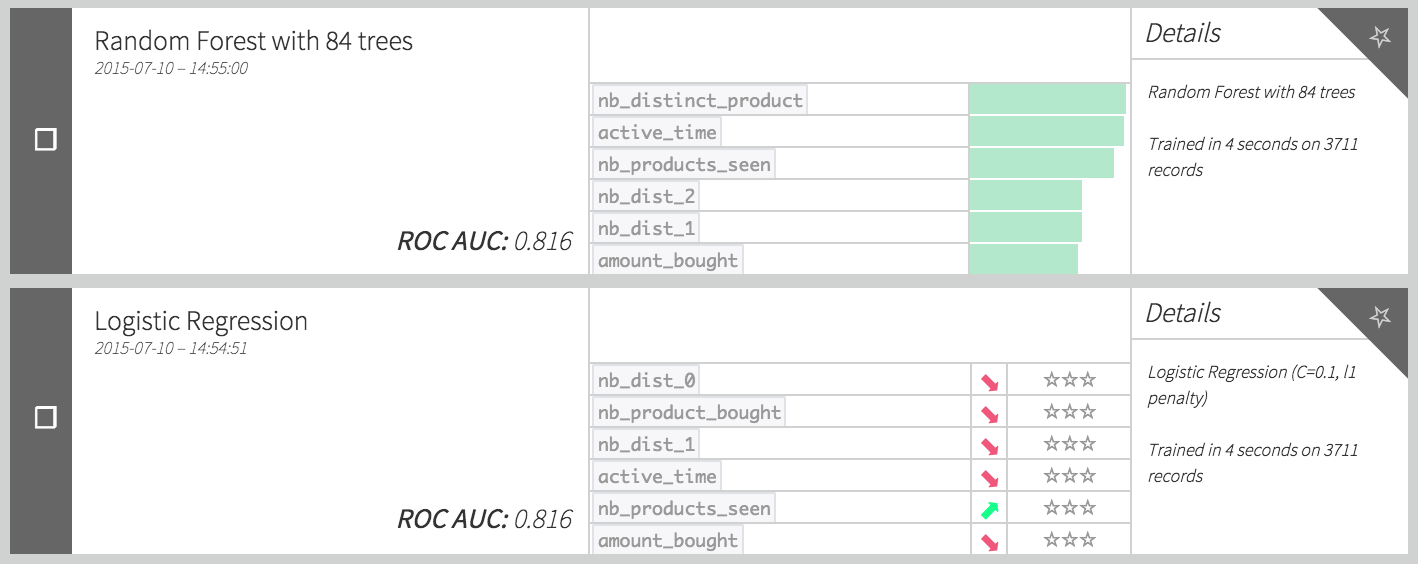
This AUC scores aren’t that bad for a first model. Let’s deploy it.