Tutorial | Distinct recipe#
Let’s practice using the Distinct recipe to remove duplicate rows in Dataiku!
Get started#
Objectives#
In this tutorial, you will:
Remove duplicates on all columns.
Check that each row in your dataset is unique.
Prerequisites#
To complete this tutorial, you’ll need the following:
Dataiku 12.0 or later.
Create the project#
To create the project:
From the Dataiku Design homepage, click + New Project > DSS tutorials > Core Designer > Distinct Recipe.
From the project homepage, click Go to Flow (or
g
+f
).
Note
You can also download the starter project from this website and import it as a zip file.
You’ll next want to build the Flow.
Click Flow Actions at the bottom right of the Flow.
Click Build all.
Keep the default settings and click Build.
Use case summary#
Let’s say we’re a financial company that uses some credit card data to detect fraudulent transactions.
The project comes with three datasets, described in the table below.
Dataset |
Description |
---|---|
tx |
Each row is a unique credit card transaction with information such as the card that was used and the merchant where the transaction was made. It also indicates whether the transaction has either been:
|
merchants |
Each row is a unique merchant with information such as the merchant’s location and category. |
cards |
Each row is a unique credit card ID with information such as the card’s activation month or the cardholder’s FICO score (a common measure of creditworthiness in the US). |
Create the Distinct recipe#
We’ll create a Distinct recipe from the tx_prepared dataset.
From the Flow, select the tx_prepared dataset and click the Actions icon (+) from the right panel to open the Actions tab.
Under the Visual Recipes section, click on Distinct.
The New distinct recipe window opens.
In the recipe dialog, change the output name to
tx_distinct
.Click Create Recipe.
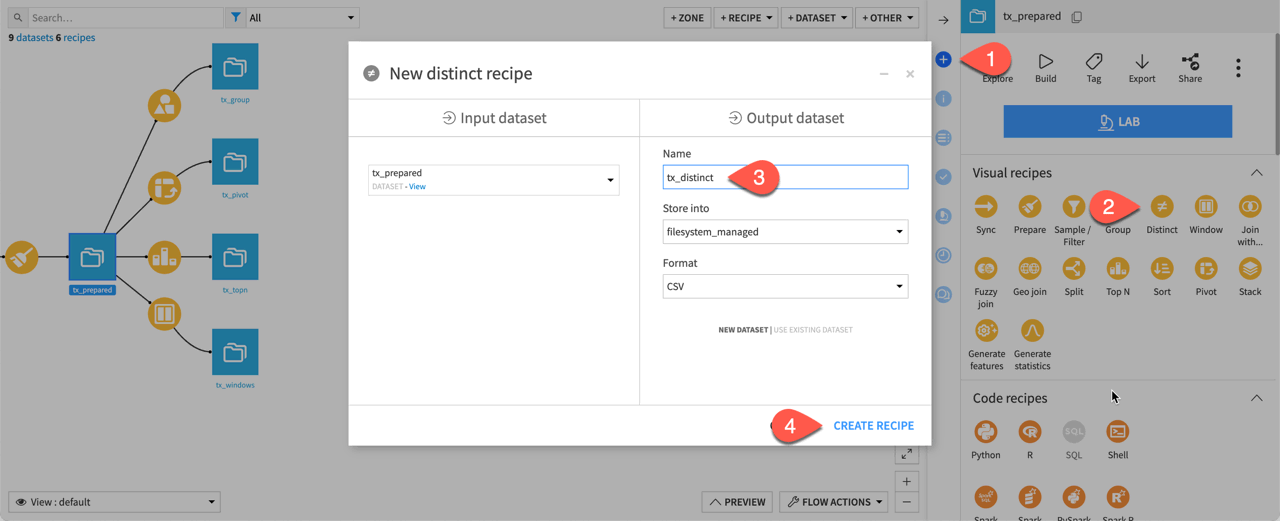
Remove duplicates on all columns#
We’ll start with the default settings to understand what happens when you remove duplicates on all columns.
Under Operation mode, keep the default selection Remove duplicates (on all columns).
Make sure Compute count of original rows for each deduplicated output row is checked under Settings.
Run the recipe and then open the output dataset.
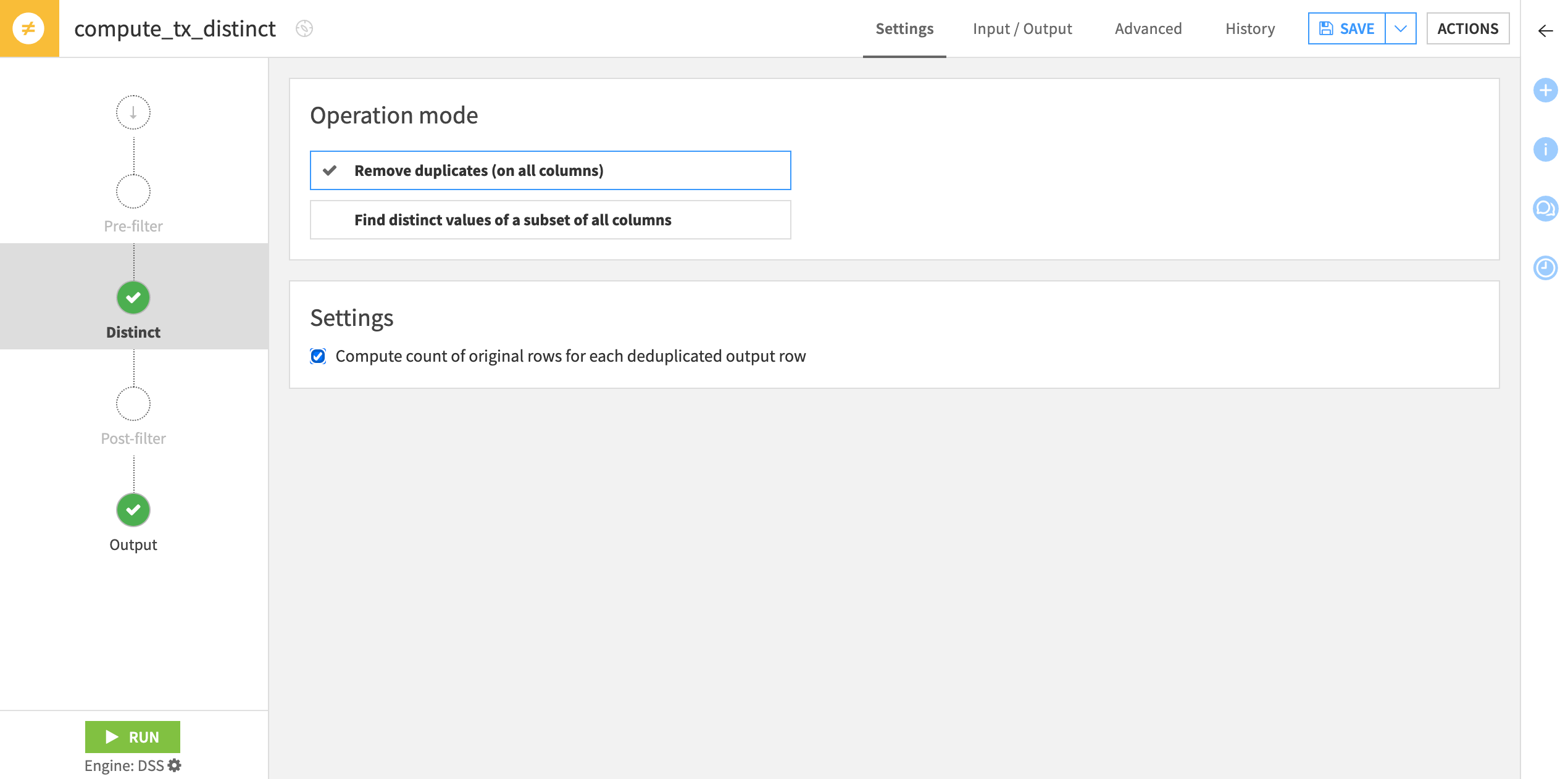
Here, you can see a new column: count. Quickly scroll and see that all counts = 1 in this sample. Let’s confirm that this is true and that there were no duplicates in the dataset.
Set the post-filter#
From the output dataset, click Parent Recipe.
Navigate to the Post-filter step of the recipe.
Toggle On the Filter tile.
Add a condition where
count > 1
.Run the recipe and then open the output dataset.
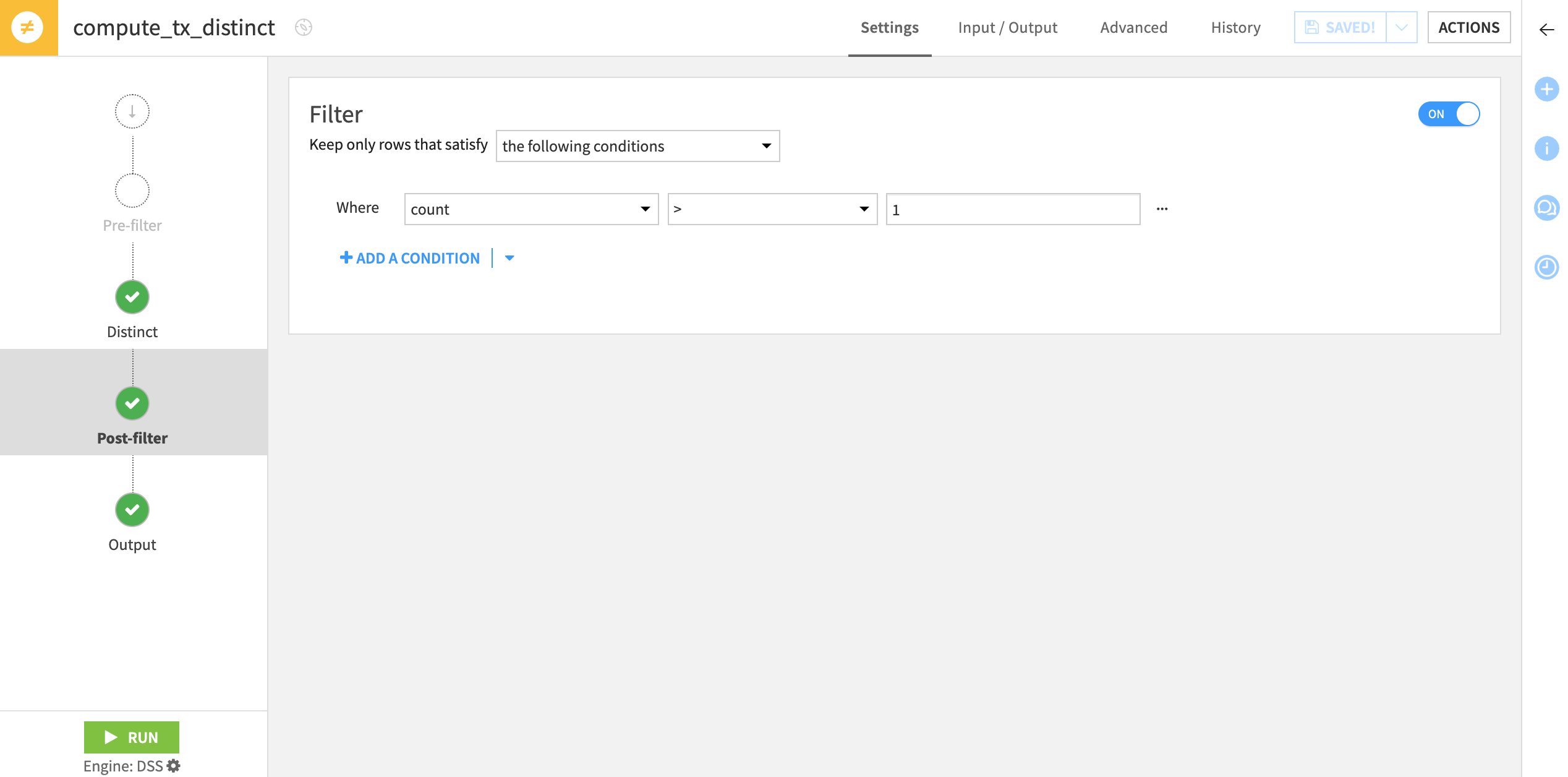
Because there are no records in the output dataset, we are now certain that there were no entirely duplicate rows in the dataset. Next, we will try to find duplicates on a subset of columns!
Find distinct values of a subset of all columns#
Let’s find out if there are any duplicate combinations of purchase_date and card_id. This will tell us if any card was used more than once in one day.
Reopen the Distinct recipe.
Under Operation mode, choose Find distinct values of a subset of all columns.
Add or keep purchase_date and card_id in the Selected columns box.
Ensure that the box Compute count of original rows for each deduplicated output row is checked.
Make sure the post-filter is still active.
Run the recipe and then open the output dataset.
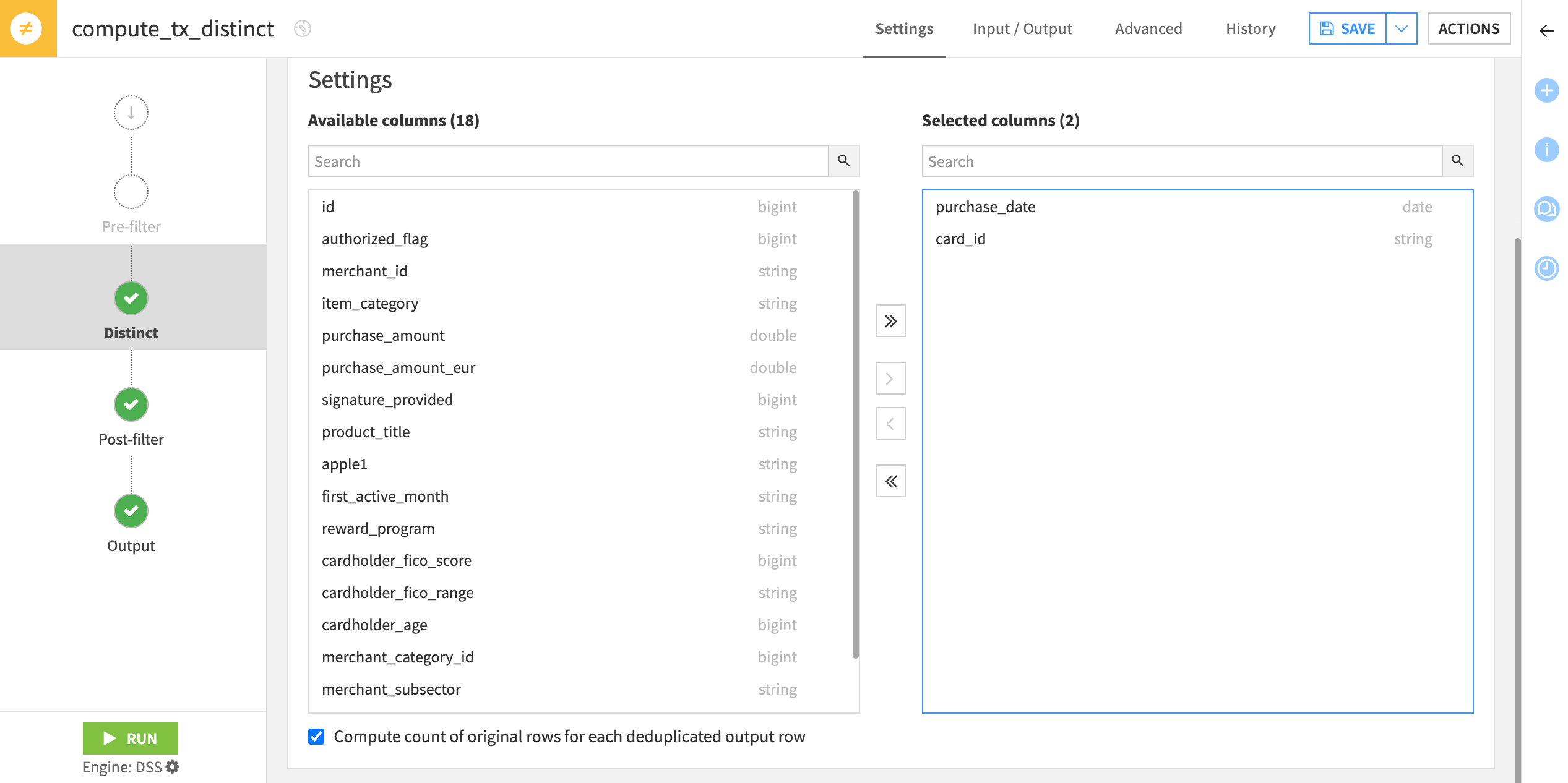
In the new tx_distinct dataset, you should see all records that have one or more duplicates across these two columns. In other words, this output shows all instances where a customer made multiple purchases using the same card on the same day.
Note
Finding duplicates is particularly useful when preparing to use the Window recipe, as it helps to confirm whether or not rows will have a unique order.
What’s next?#
You just practiced using the Distinct recipe to find duplicates in your data.
See also
For more information on this recipe, see also the Distinct recipe article in the reference documentation.
To try out more visual recipes, visit our page on Visual Recipes!