Concept | Model transparency#
Explainable AI requires observation of how data is prepared, how it is modeled, and what the expected use of an AI system is. These insights are critical for building trust between your analytics and stakeholders, including operations teams, business users, and end consumers. Explainability also supports better data-driven decision making.
Dataiku offers various features that support explainability and can be customized at different levels of detail that make sense for different consumers of an AI system.
Creating the right kind of reporting and feedback mechanisms helps further goals around transparency and prevents biases from emerging downstream in AI pipelines.
Note
For a general overview of Dataiku’s native Explainable AI capabilities, review this article.
Reporting and model transparency#
Even a completely fair model can be deployed in an unsafe way that causes unforeseen harms. Therefore, we must have ample reporting on all models, regardless of perceived fairness, to reference when investigating or addressing any harms.
Providing transparent and clear reporting to end consumers is a good way to prevent deployment bias, but the kind of reporting needed will depend on the kind of end user.
When it comes to putting analytics and AI projects into production, you will need to think about three kinds of consumers: operations, business users, and affected clients. Each of these consumers will have different questions about a model or analysis either before or after it is in production.
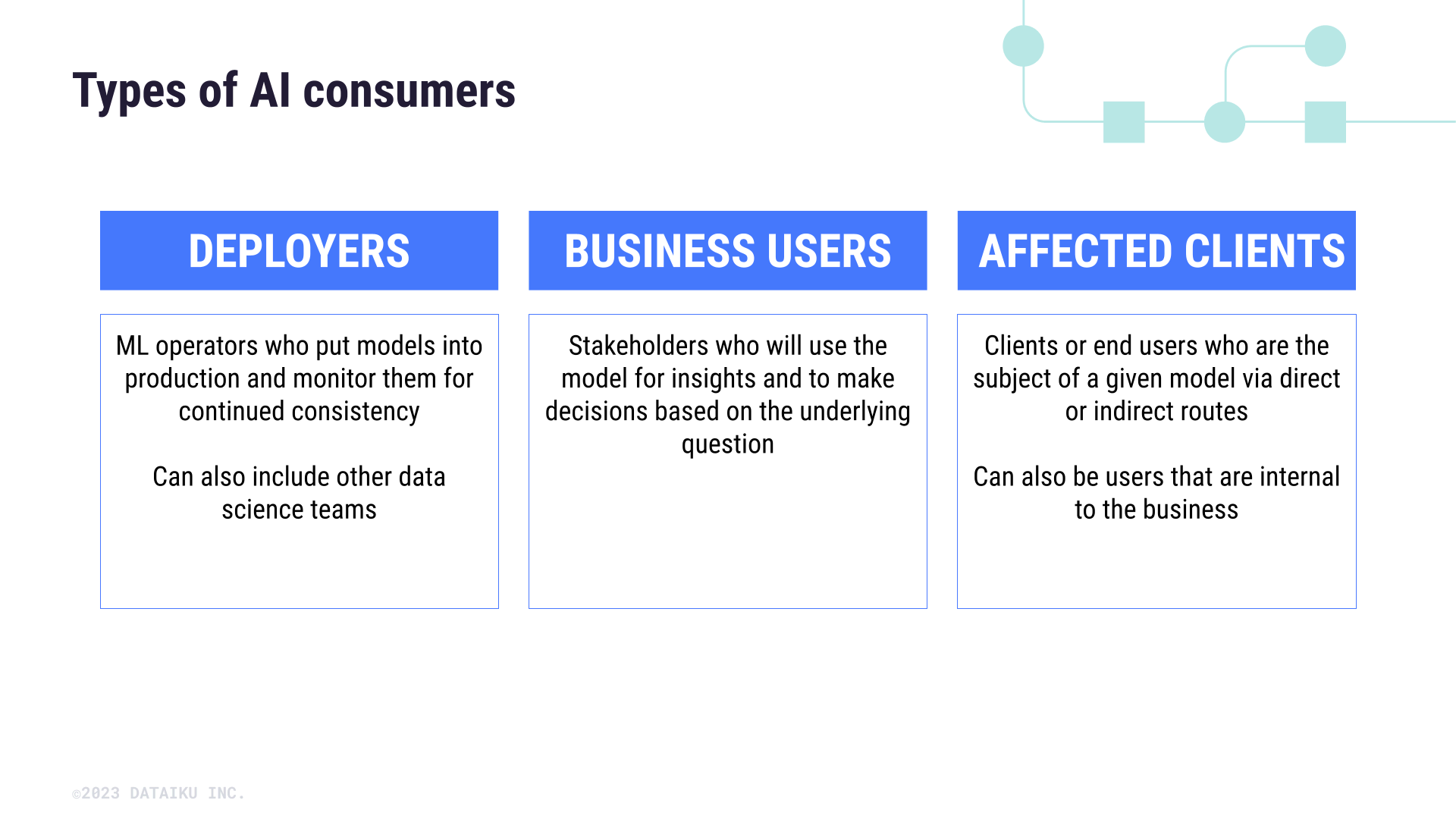
Deployers need to know:
The thresholds for reliability and fairness for a model.
The kind of failures that warrant stopping a model in production.
Who is accountable for assessing AI risks, such as a governance manager or review committee, to direct potential issues to the right teams early on.
Which downstream units should have access to the outputs of models, or what context models were built for.
Business users are concerned with understanding:
The kind of data used to build an analysis or a model.
The type of information used to understand underlying biases.
Any mitigation steps that were taken during the project build.
The exact questions that the analysis or model can be used to answer and what kind of outcomes a model has been optimized for.
End clients (the people directly affected by the outputs of a model) should be aware of:
When their access to or the quality of a service is determined by an algorithm.
Why the model predicts a certain outcome.
The overall distributions of the data used to train the model.
Ways to contest or counter the results of a model via a feedback mechanism.
An additional type of reporting is often required for auditors and regulators. These reports should cover the entire lifecycle of development from data cleaning to final model selection.