Solution | News Sentiment Stock Alert System#
Overview#
Business case#
Traders, equity analysts and portfolio managers have to leverage an ever growing stock of information to fuel their company analysis. Of vital interest is knowing:
What stocks are most likely to move based on current news sentiment?
What are the underlying news events driving volatility for a specific ticker?
What historical insights can one gain through systematic analysis of past news events?
Automatic anomaly detection removes the need for costly or small scale labelled datasets. It avoids unfocused manual review that’s costly and inefficient, and it works alongside purely automatic trading responses based on news sentiment, which may miss important opportunities.
An easy-to-use interface allows for immediate insights, rapid drill-down, and deeper analysis of trends, all with a few clicks. Flexible design allows for enhancement or customization to meet a team of firms specific needs.
Installation#
From the Design homepage of a Dataiku instance connected to the internet, click + Dataiku Solutions.
Search for and select News Sentiment Stock Alert System.
If needed, change the folder into which the Solution will be installed, and click Install.
Follow the modal to either install the technical prerequisites below or request an admin to do it for you.
From the Design homepage of a Dataiku instance connected to the internet, click + New Project.
Select Dataiku Solutions.
Search for and select News Sentiment Stock Alert System.
Follow the modal to either install the technical prerequisites below or request an admin to do it for you.
Note
Alternatively, download the Solution’s .zip project file, and import it to your Dataiku instance as a new project.
Technical requirements#
To leverage this Solution, you must meet the following requirements:
Have access to a Dataiku 12.0+* instance.
A Python 3.8 code environment named
solution_stock-alert-system
with the following required packages:
scikit-learn>=1.0,<1.1
dash==2.7
dash_bootstrap_components==1.2.1
tzlocal==4.2
plotly==5.13.0
Data requirements#
The Dataiku Flow is built using publicly available data on stock prices and the news. The Solution itself doesn’t contain any direction connection to an external data source. Rather, input datasets should be separately retrieved and linked to the data sources necessary for the project to work.
Dataset |
Description |
---|---|
tickers_information |
Contains 1 row per ticker with information about the sector of the stock. |
stock_prices_all |
Contains historical stock prices for all tickers contained in tickers_information. One row should correspond to one day for one ticker. |
news_data |
Historical news with the tickers labeled. Each row contains an individual piece of news. The time period of this dataset should match stock_prices_all. |
news_today |
Contains latest news with the same format as for historical news. |
Workflow overview#
You can follow along with the sample project in the Dataiku gallery.
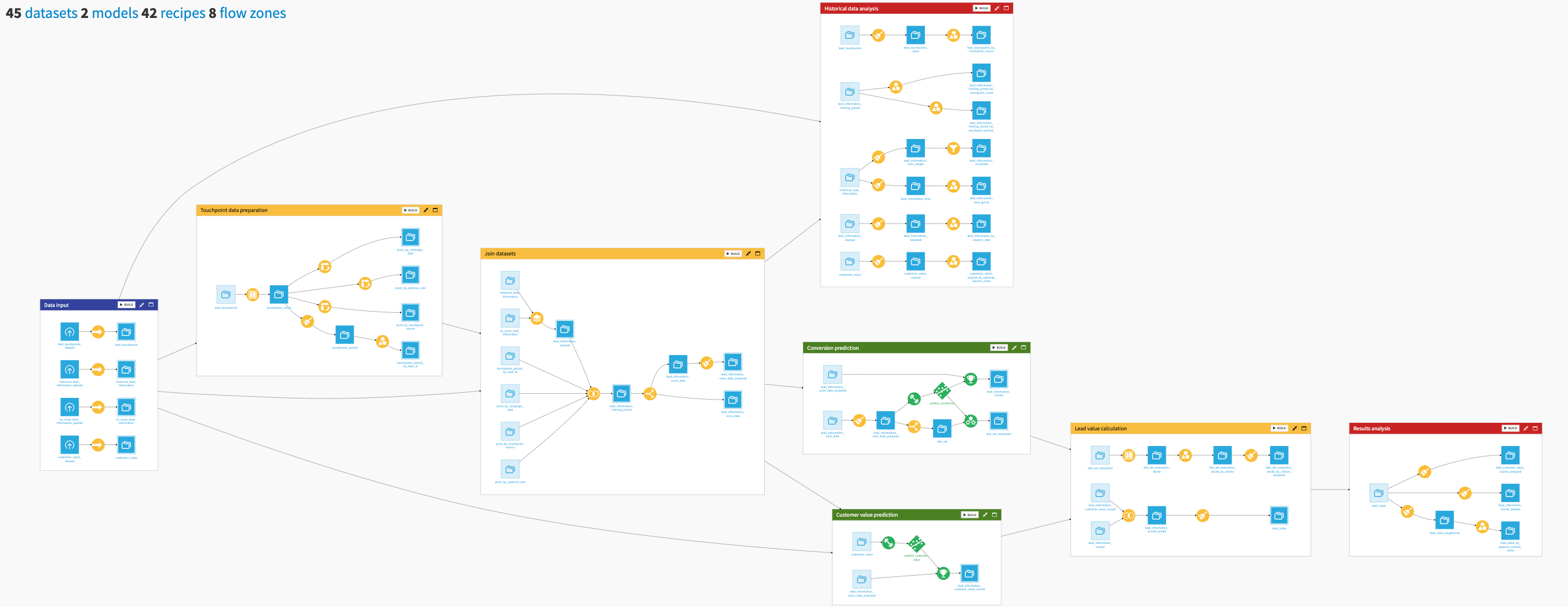
The project has the following high level steps:
Input the list of tickers and news source on which the analysis will be done via Project Setup.
Retrieve stock prices and news into partitioned datasets.
Analyze the stock prices to detect anomalies.
Train a model to predict stock price anomalies using the news.
Score real time data to produce risk scores and impact rankings.
Visualize data using a pre-built Webapp and Dashboard insights.
Walkthrough#
Note
In addition to reading this document, it’s recommended to read the wiki of the project before beginning to get a deeper technical understanding of how this Solution was created and more detailed explanations of Solution-specific vocabulary.
Tailor the alert system to your interests#
By default the project already contains tickers for the S&P 500 stocks and news data from 2022. The project can be overridden by utilizing the built-in Project Setup which you can access from the homepage of the project. The Project Setup enables users to connect their own input data and rebuild the entire Flow with this new data. Real time risk scoring can also be manually run from the Project Setup interface. Changing the connected datasets will impact the Input Data Flow zone.
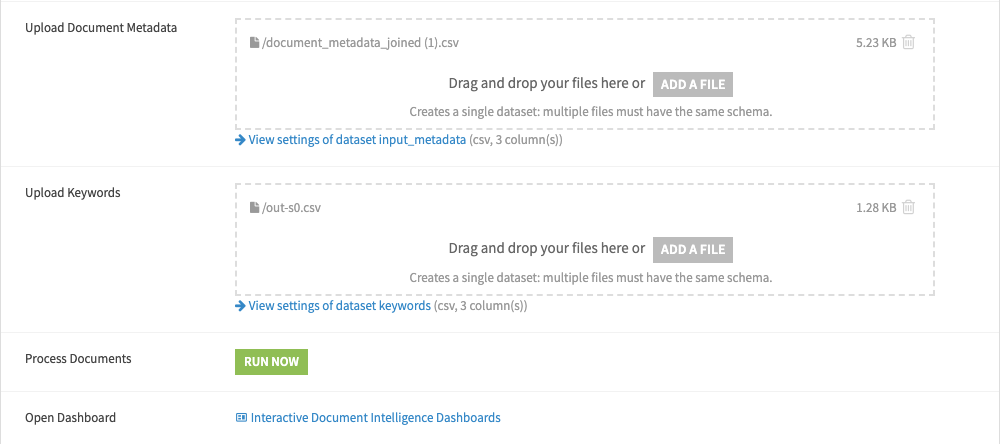
Detect anomalies in stock prices#
One can define an anomaly as a move that’s peculiar with regards to the historical moves of a stock. The Anomaly Detection section of the wiki gives further detail on what’s considered peculiar stock behavior, stock clustering, and the anomaly detection algorithm in use.
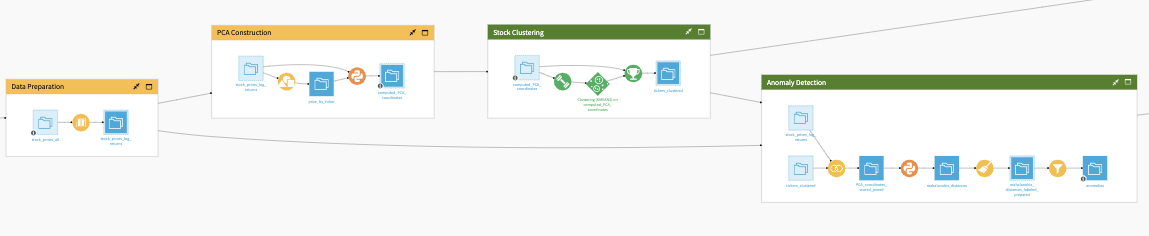
The analysis to detect anomalies consists of four parts:
Header |
Header |
---|---|
Data Preparation |
Is where stock prices are processed to compute the log returns. |
PCA Construction |
Includes a python recipe that takes the data with the log returned from the previous zone, computes the covariance matrix and then runs the Principal Components Analysis (PCA). The recipe outputs the coordinates on the first four Principal Components for each stock. |
Stock Clustering |
Takes the PCA coordinates and runs clustering on the stocks using a K-Means algorithm. We chose the algorithm and cluster number (8) used for this Solution for its simplicity, but you could try other algorithms for more in depth cluster analysis. |
Anomaly Detection |
Partitions the initial log return dataset using the clusters output from the Stock Clustering zone so that anomaly detection can run on each partition independently. Anomaly detection is based off of Mahalanobis Distance computations run within a Python recipe and labeled based on a predefined threshold. |
Train predictive models and score real-time data#
The Cross Data Analysis zone joins and further cleans the processed news data and cleaned stock pricing data. The combined dataset is then used to train a logistic regression model to detect anomalies.
The Real Time Alert Flow zone uses the final model to score real time data to produce a risk score for each stock today. Additionally, this zone ranks individuals news events with regards to the impact they have on related stock movement.
The Visualization zone also scores past data to enable users to investigate past news events with large impacts on stock movements within the webapp interface.
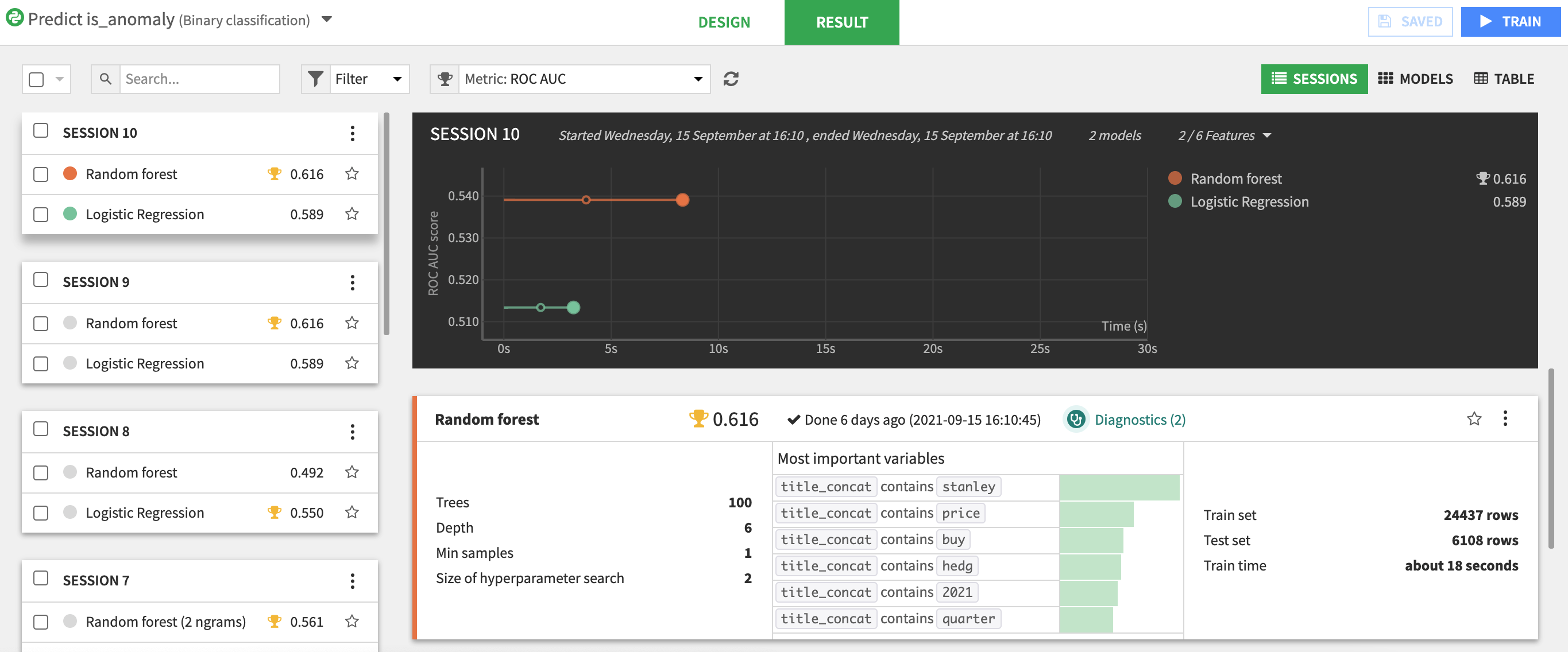
The Solution includes two scenarios to automate the Flow and keep it up to date with real-time data. The Overnight Batch scenario adds the previous day’s data and updates the models. Real Time Risk Scoring retrieves the most recent news, processes, and scores them to feed real-time investigation of stocks from the webapp. You can make additional configurations to these scenarios to send reports.
Investigate the impact of news on stock prices#
The Solution dashboard to consume the results of the analysis. The webapp, contained in the first page of the dashboard, contains four tabs:
Tab |
Description |
---|---|
Real Time News Scoring |
Gives, in real time, the volatility score per stock and allows users to browse through news of the day. Each row of the first table of stocks is selectable to filter a second table of news articles that impact a particular stock. The whole view is reset at midnight UTC but will re-populate throughout the day. ![]() |
Case Study |
Makes it possible to navigate through past anomalies detected by the algorithm and visualize the price evolution and the news around the anomaly. Once again, the first table consists of selectable rows that will update a graph of the price of the stock around an anomalous event and a table showing news leading up to and following the event. |
Historical Prices Anomaly Detection |
Presents a visualization of the historical prices of a given stock with an adjustable time frame. |
Historical New Scoring |
Enables users to browse through the full news dataset that has been processed in the project for each stock. |
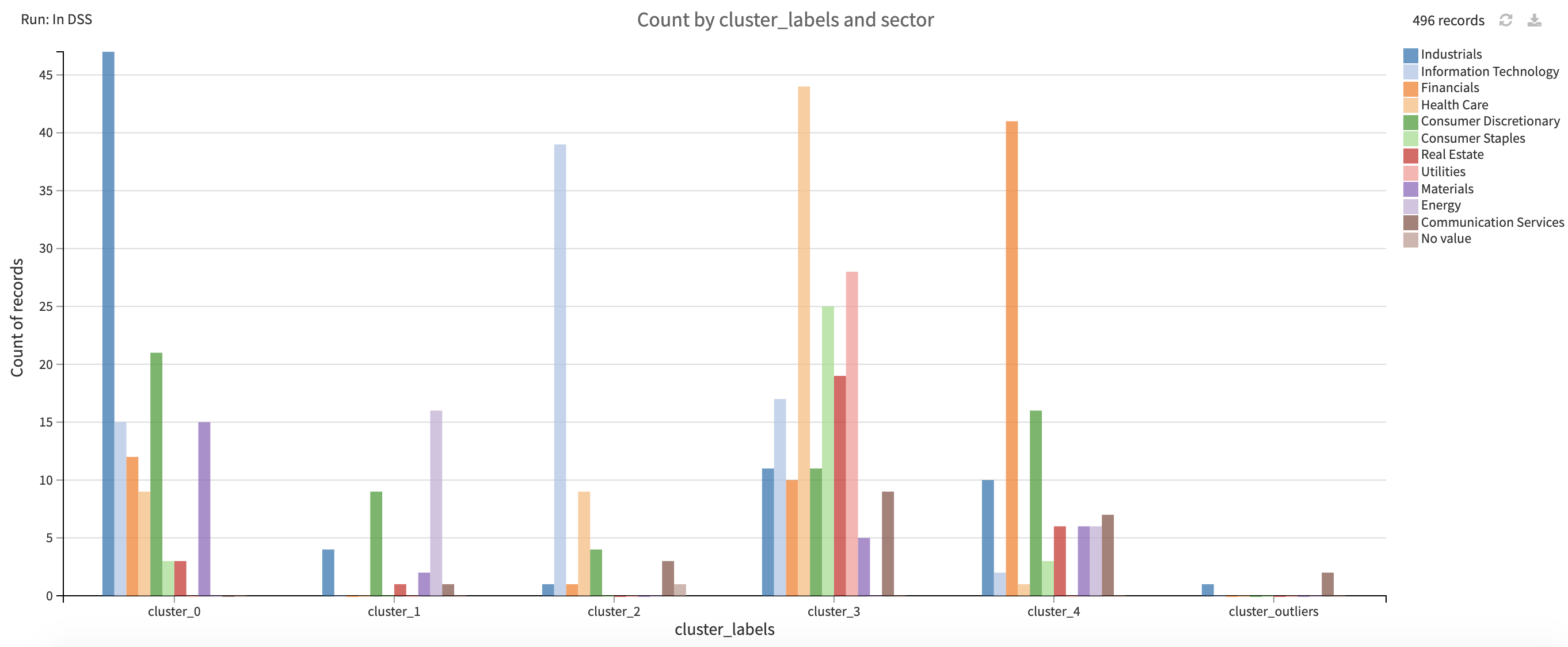
The dashboard contains four additional tabs (Alerts, Model, Anomalies, and Clusters) to allow users to visualize:
The real time view of the scores by stock
A report on the New Scoring Model
Insights into the anomalies detected
Visual cluster analysis
Reproducing these processes with minimal effort for your data#
The intent of this project is to enable traders, equity analysts, and portfolio managers to understand how they can use Dataiku to ever growing stock of information: to know
What stocks are most likely to move based on current news sentiment?
What are the underlying news events driving volatility for a specific ticker?
What historical insights can one gain through systematic analysis of past news events.
By creating a singular Solution that can benefit and influence the decisions of a variety of teams in a single organization, you can design smarter and more holistic strategies to reduce costs, avoid unfocused manual review, and work alongside automatic trading responses.
This documentation has provided several suggestions on how to derive value from this Solution. Ultimately however, the “best” approach will depend on your specific needs and data. If you’re interested in adapting this project to the specific goals and needs of your organization, Dataiku offers roll-out and customization services on demand.